This issue highlights (underlooked) nuances across AI:
Lower switching costs
Open source language models
Small language models
Taken together *these* nuances are favorable to disruptors and are accessible at a smaller scale.
That said, winning categories always comes back to GTM, and these nuances only distantly touch GTM.
Creandum on AI - Lower Switching Costs
While the whole “AI in B2B SaaS: Beyond Vertical and into the Horizon(tal)” post from Creandum (a European early-stage VC firm) is worth reading, I wanted to call out this point:
Lower Switching Costs:
“With new advancements in AI it is now also significantly easier to take unstructured data that sits in PDFs or other static formats and turn them into structured data. This evolution has a direct impact on reducing switching costs for enterprises. Whereas in previous platform shifts, data ingestion and migration were cumbersome and served as a substantial moat for incumbents, suddenly companies can find it easier to transition between software solutions without the fear of data loss or extensive downtimes.” (my emphasis added in bold)
Tidemark on AI - Open Source LLMs
Dave Yuan’s “AI: Extinction or Evolution? The Opportunity for Workflow Software & Vertical SaaS” highlights how open-source might be underappreciated:
“The Leapfrog Opportunity: Function-Specific-Training of Open Source LLMs: Current conventional wisdom is that it’s too expensive to build or train your own LLM, and while building your own from scratch is cost-prohibitive and constrained by GPU, open-source LLMs are too far behind and talent is too scarce. Most investors will tell you to suck it up and pay your OpenAI tax.
We think that open-source is going to play a far more important role than people realize. We’re hearing that LlamaX is already reaching GPT3.5 power. With this in mind, as you initially experiment with API access LLMs like GPT4, run concurrent tests with open source alternatives. You’ll find that you can get most of the way there with relatively little effort.” (my emphasis added in bold)
Dave’s take is proving prescient: a fresh The Information story (gift link here) highlights rising open source language model use - here with Salesforce:
“Earlier this year the sales software firm was relying on OpenAI’s GPT-4 large language models for tools that automatically draft emails or summarize meetings. Salesforce still uses OpenAI but is trying to power more of its AI services with open-source models as well as those it has developed in-house, both of which can be less expensive, said Salesforce’s senior vice president of AI, Jayesh Govindarajan.”
With open source models also being adopted by start-ups at similar quality levels:
For instance, Pete Hunt, founder and CEO of developer tools startup Dagster, recently started Summarize.tech, which automatically summarizes the contents of videos or audio. Hunt was using OpenAI’s GPT-3.5 model to power the service, which has roughly 200,000 monthly users, but recently switched to Mistral-7B-Instruct, an open-source model. As a result, Hunt’s service went from racking up around $2,000 per month on OpenAI costs to less than $1,000 per month, and users haven’t complained about any change in quality, he said.
Air Street on AI - Small Language Models
Air Street’s “State of AI 2023” included a summary of exploratory research by Microsoft on small language models:
“When small language models (SLMs) are trained with very specialized and curated datasets, they can rival models which are 50x larger. They also find these models’ neurons are more interpretable.”
This reminded me of this quote1 on “golden data” from
:“Quantity has a quality that’s all its own, but when it comes to training data, the converse is also true. ‘Data quality scales better than data size’: above a certain corpus size, the ROI from improving quality almost always outweighs that from increasing coverage. This suggests that golden data — data of exceptional quality for a given use case — is, well, golden.”
Cloud Returns Podcast Episode
First, an additional quote from Creandum on AI Wow Experiences:
“From a first principles perspective, what really is a SaaS application? It's data and workflows. Now, with chat-based interfaces and agents, the way users interact with data and how these workflows are carried out is changing fundamentally. This is leading to the emergence of software categories where the dominant feature will be AI, with companies wowing users from the get-go by delivering a previously unthinkable product experience. With that shift, new opportunities in horizontal software emerge.”
This Creandum quote directly aligns with Nick Tippman’s podcast comments on AI creating “10x experiences” that unlock previously inaccessible, software-avoidant vertical SaaS markets: EPISODE HIGHLIGHT
In the rest of the episode, we cover his vertical SaaS investment criteria, founder-market fit, the impact of APIs, and much more:
Subscribe To “Cloud Returns” (our cloud investing podcast) On: Spotify | Apple Podcasts | All Other Platforms
Curated Content
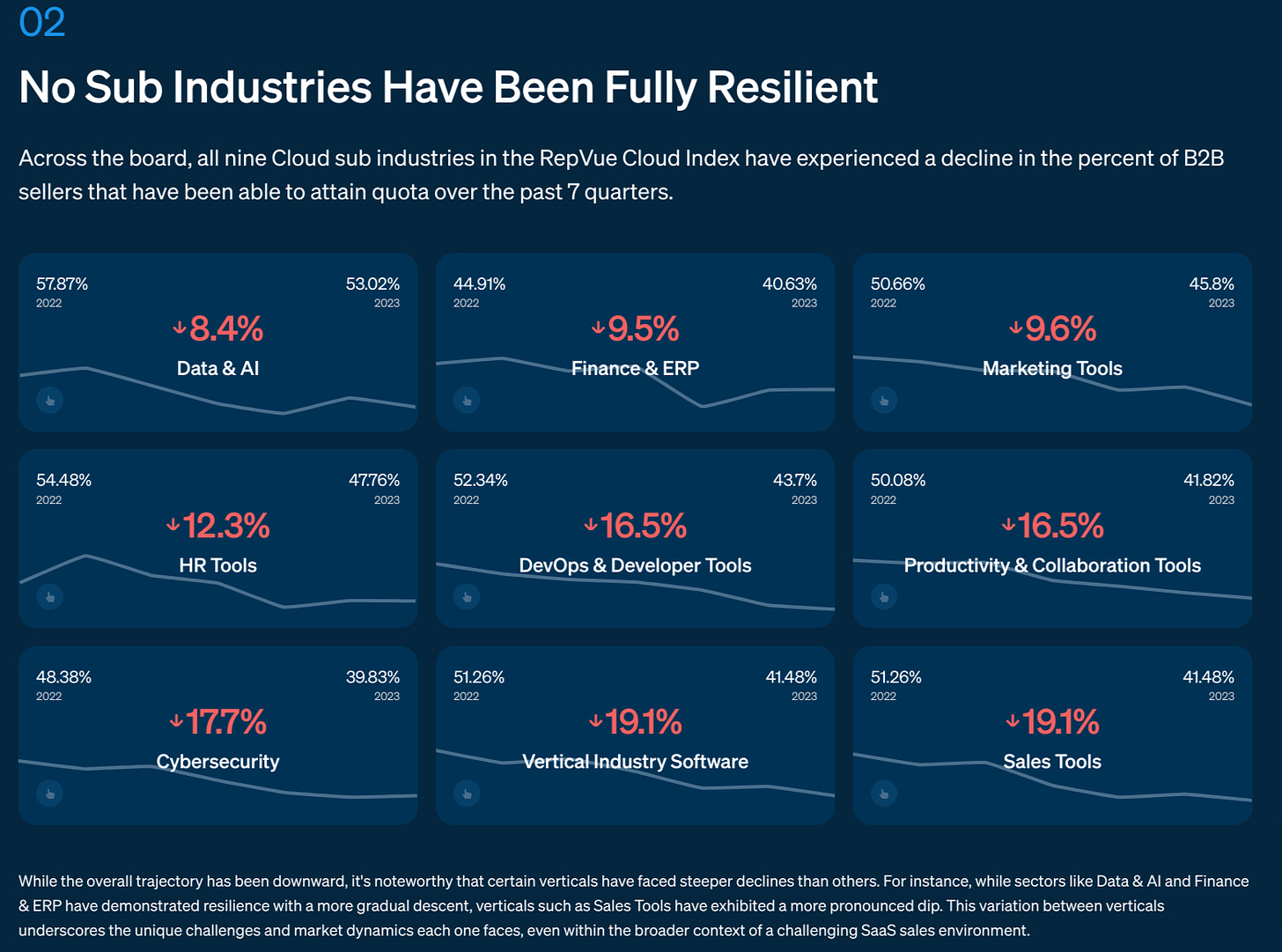
RepVue debuted their Cloud Index - new + valuable data points include category level trends, sales cycle lengths, quota attainment by deal size, and average deal sizes. More in my thread.
Morgan Stanley CIO Survey - Q3 2023 - my thread summary:
Overall IT spend trends stable + in-line with consensus
AI implementations weighted to *2H 2024*
63% of vendors implementing notable price increases
CIO #1 response to price in survey = vendor consolidation
A solid “2023 State of DevOps Report” from Google Cloud + DORA
The 73% gross retention rate for vendors WITHOUT customer success functions captured my attention - from a Gainsight / Benchmarkit study:
Initially included in my “AI In Finance + The Enterprise” post.